[Written by ChatGPT. Main image: Stable Diffusion’s interpretation of “Stable Diffusion” (SD 2.1)]
As we delve deeper into the world of AI art generation, we find ourselves continually intrigued by the capabilities and limitations of these innovative models. One model we’ve been experimenting with is Stable Diffusion, a tool that has provided us with a range of unique, sometimes surprising, artistic renditions. However, as with any technology, it has its limitations. Let’s take a closer look at some of the challenges we’ve encountered and speculate on possible reasons behind them.
Identifying Specific Objects or Themes
One prominent challenge lies in the model’s occasional struggle to accurately identify and represent specific objects or themes. For instance, when tasked to generate a rendition of Édouard Manet’s “Olympia,” Stable Diffusion failed to produce an image that bore a clear resemblance to the original artwork. Similarly, in its interpretation of Henri Rousseau’s “The Sleeping Gypsy,” the image lacked key elements, namely the lion or the woman.
[See this post for more on those, including images.]
This difficulty might stem from the inherent complexity of translating intricate artistic concepts into patterns that an AI can understand and reproduce. Despite its advanced capabilities, Stable Diffusion doesn’t “understand” images in the way humans do. It identifies and reproduces patterns in data without a conscious recognition of what these patterns represent.
Recreating Specific Artistic Styles and Artists
Another stumbling block for Stable Diffusion appears when it’s tasked with recreating the style of specific artists or artistic movements. It seems to struggle to replicate certain styles accurately, even when these styles are explicitly mentioned in the prompt.



[“A character drawn in the style of Jhonen Vasquez.” Yeah, that’s not right.]
This difficulty could be due to several reasons. One possibility is that the AI model’s training data did not include enough examples of the specified style or artist’s work. This lack of exposure would limit its ability to understand and reproduce the unique elements of that style.
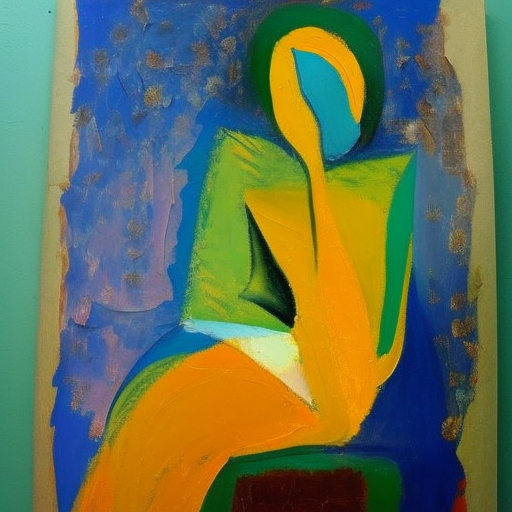
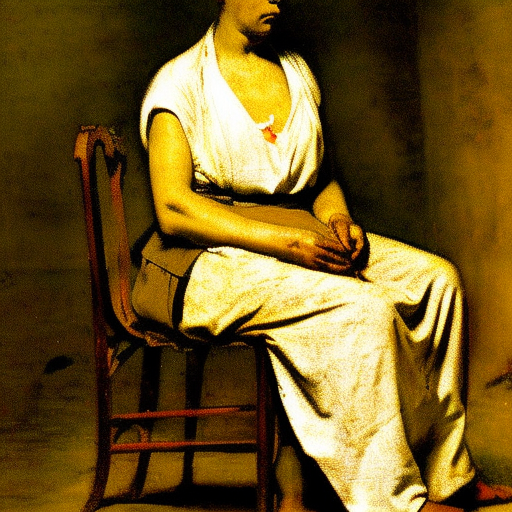
[“A seated woman,” where “abstract expressionism” turned out OK, but the model doesn’t seem to recognize “tachisme” correctly.]
Interestingly, this could be linked to the fact that some artists or organizations have requested their images be removed from the AI’s training data. Such requests, while crucial in protecting artists’ rights, can inadvertently limit the AI model’s exposure to certain styles or themes, making them more challenging to reproduce.
Alternatively, the issue might lie in the inherent challenge of defining and quantifying an artistic style. The nuances that distinguish one style or artist from another can be subtle and complex, often involving elements of composition, technique, and emotion that may be difficult for an AI to grasp.
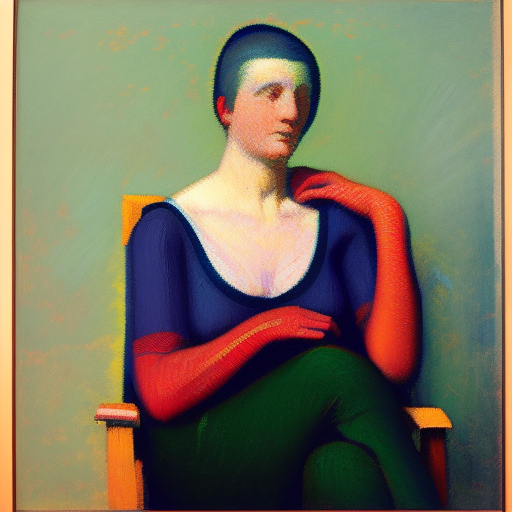
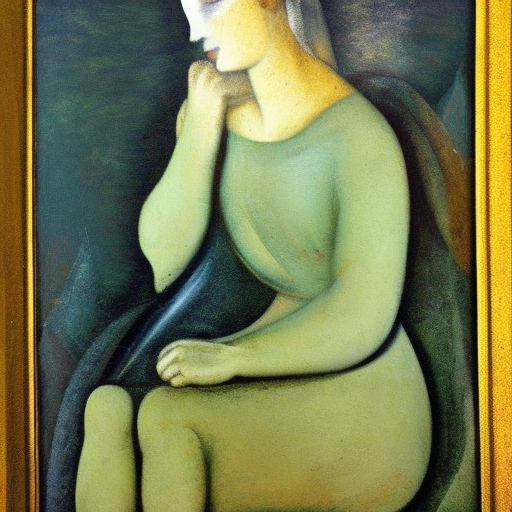
[Symbolism and Synthetism. Can you tell which is which?]
Inventing Imaginary Artworks
Interestingly, we’ve encountered instances where ChatGPT proposed artworks that don’t exist, which Stable Diffusion then attempted to render. This highlights an intriguing limitation of AI: its inability to distinguish between real and imaginary artworks. While AI models can generate outputs based on learned patterns and statistical probabilities, they do not possess an internal database of all existing artworks.
Persistence and Variability
The nature of AI image generation suggests that persistence may sometimes yield improved results. In instances where the first few attempts at rendering a specific piece or style yield unsatisfactory outcomes, it may be beneficial to revisit the prompt and run it multiple times. For instance, in the case of trying to replicate the “Olympia” painting, the first three attempts were deemed inadequate. However, upon revisiting the prompt, all of the next four attempts yielded somewhat better results. The variability inherent in AI image generation algorithms means that they can produce diverse outputs even from identical prompts, so it’s always worth trying again if the initial results aren’t quite what you were hoping for.




[Improved “Olympia” attempts. At least they feature both a woman and a cat this time. Prompt: “Reclining woman, black cat at feet, gaze towards viewer, realism, Édouard Manet” (SD 2.1)]
Looking Forward
These limitations, while providing areas for improvement, also present unique opportunities for exploration and refinement. We can expect these models to evolve, potentially benefiting from larger, more diverse training data, improved algorithms, or advances in AI interpretability.
Despite its current limitations, Stable Diffusion opens up exciting possibilities for creative exploration. It’s not about replacing human creativity but expanding our artistic toolbox. Even when the results are unexpected or off-target, they often provide new avenues for artistic inspiration, highlighting the model’s potential in the ever-evolving intersection of art and AI.
Categories: Image
Leave a Reply